Remote Sensing to Facilitate Intervention Scaling
Given that our aquatic vegetation removal intervention offers a mutually beneficial innovation for several societal challenges and there was some level of interest in adopting the intervention, we began developing a scaling plan. We hypothesized that we could use satellite imagery to identify snail habitat and thus potential schistosomiasis hotspots, which would then facilitate targeting our intervention to where it is needed most. The application of a deep learning fully convolutional neural network, allowed us to reliably discriminate submerged vegetation from emergent vegetation, open water, and land (segmentation accuracy for the submerged vegetation was 97.0% for the training set and 84.0% for the validation set, Fig. 1, from Rohr et al. 2023). This suggests that there is considerable potential to use remote sensing to facilitate targeting and scaling of this intervention. Scaling of this intervention could also be further assisted by several next steps, such as investigations of (i) vegetation removal intervals, spatial scales, compliance, and mechanization, (ii) other economic uses of the vegetation, and (iii) the efficacy of the intervention in other parts of the world.
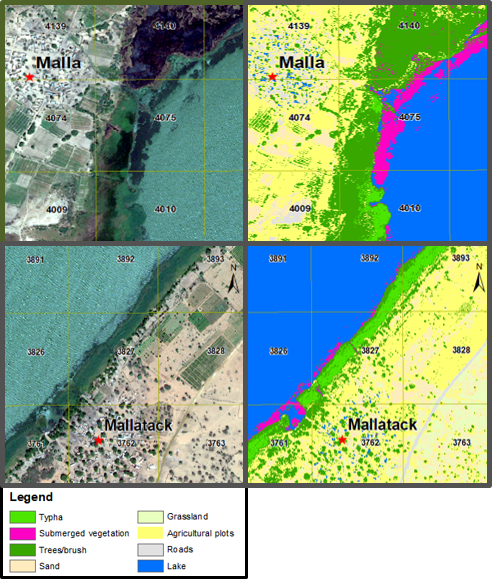
For more information, see Rohr et al. 2023.